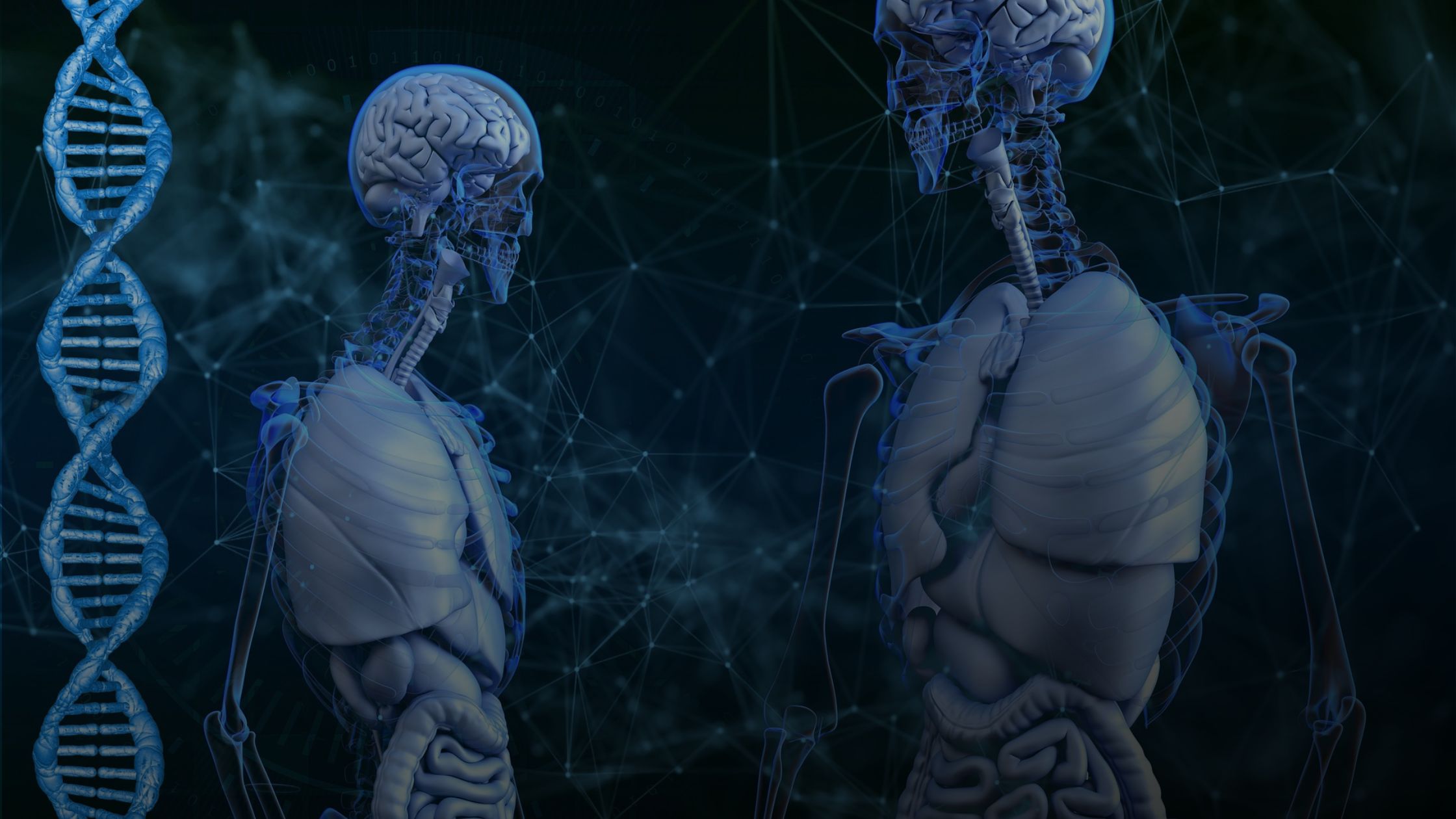
- Rahana A Kadir
- 4
Machine Learning in Medical: Applications, Pros & Cons
Machine learning (ML) is rapidly transforming the medical and healthcare industries by enabling healthcare professionals to analyze large amounts of data and make more informed decisions about patient care.
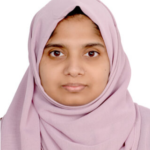
Rahana A Kadir
February 27, 2023 – 4 min read
Machine learning (ML) is an artificial intelligence subfield that involves the development of algorithms that can learn from data. Thus, machines can “understand” complex tasks and carry out desired actions without being explicitly programmed.
A variety of industries use ML, including agriculture, retail, education, healthcare, and finance. Why? Because machine learning applications help them to improve the:
- accuracy and speed of decision-making processes
- prediction of outcomes for future events
- effectiveness of data analysis
So, machine learning is gaining popularity across industries and verticals. Talking of the medical field, ML has already stepped in.
You will find that medical diagnoses and treatments are becoming more accurate. We also have automated health records management. Robots are assisting surgeons in performing surgeries, and much more.
Since ML algorithms can identify patterns in patient data that may not be apparent with classic methods, machine learning is on its way to revolutionizing the medical and healthcare industry.
In this post, we will discuss:
- Various machine-learning applications in the medical and healthcare fields
- Advantages and disadvantages of using machine learning in the medical and healthcare fields
Machine Learning Applications in Healthcare
Medical machine learning had come a long way since its inception in the early 1990s when it was used for the diagnosis of diseases. Today, it helps medical and healthcare professionals in many ways.
1. Predicting treatment outcomes
The most significant machine learning application is predicting treatment outcomes. ML algorithms are capable of forecasting how well a patient will respond to a specific treatment.
Several models, including Cox regression, logistic regression, and linear regression, help achieve this.
In some cases, machine learning in conjunction with other medical techniques such as biometric data is used. This is done to get a better idea of how the patient will react to treatment.
2. Task automation
Task automation in the medical and healthcare industry improves overall efficiency. It reduces the time spent on specific tasks and makes it easier for medical professionals to execute their duties.
3. Identifying diseases and diagnosis
Machine learning helps in diagnosing diseases and ailments that are otherwise difficult to identify.
4. Wearable healthcare devices
Medical wearables monitor a person’s health and display any warnings when something unusual or unlikely is observed. Even smartwatches use ML to detect the basic medical condition of the person who wears them.
5. Drug Discovery and Manufacturing
ML algorithms help in drug discovery and manufacturing. Using unsupervised learning, they can identify patterns in data without making predictions.
It includes research and development technologies like next-generation DNA sequencing and precision medicine. Besides, machine learning can even aid in the discovery of alternative treatment pathways for multifactorial diseases.
6. Data Collection
Machine learning helps in live medical and healthcare data collection. Using the database, medical practitioners can access the full range of information about a patient.
Many tech behemoths, including Apple, IBM, and others, are utilizing machine learning to collect patient data. There are various advanced electronic devices employed for this purpose.
7. Robotic surgery
Robotic surgery is a breakthrough application of machine learning in the medical field. The trained robots know how to perform surgery and assist doctors in procedures.
It is further divided into four subcategories:
- surgical skill evaluation
- surgical workflow modeling
- automatic suturing
- robotic surgical material improvements
Disadvantages of Machine Learning in the Medical Field
The use of machine learning in medicine has some obvious advantages. For starters, the ability to use data to diagnose and treat patients has the potential to save lives. Healthcare wearables can alert people beforehand if they notice any abnormal readings. The discovery and development of life-saving drugs and medicines take lesser time.
However, there are some drawbacks to using machine learning in this field.
1. Are often biased
The major problem with machine learning algorithms is that they are often biased. They are more likely to produce results that support the hypotheses that were used to train them. And so, they don’t understand and reflect the true nature of the data. This can lead to inaccurate diagnoses and treatments.
2. Needs human surveillance
Machine learning is way too advanced than it was a decade ago. It can do any task with perfection but still, it needs human surveillance. Since medical and healthcare deal with the lives of people, human surveillance is more necessary. In case of any fault in the robots or an error in the algorithm occurs, human beings have to take action.
3. Inaccuracies may still exist
Medical AI relies heavily on diagnosis data from millions of documented cases. Misdiagnosis is entirely possible when there is little data on specific illnesses, demographics, or environmental factors. So, even though algorithms can speed up tasks, monitoring is necessary for verification. It is especially important when it comes to prescribing specific medications.
4. May cause unemployment
According to a World Economic Forum report from 2018, AI will generate 58 million new jobs by 2022. However, the same study predicts that AI will displace or destroy 75 million jobs in the same year. The reason? As AI gets more integrated, roles that need repetitive tasks will become obsolete.
Future of Machine Learning in Medical & Healthcare
The future of artificial intelligence in the medical and healthcare industry is bright. It will bring to life truly proactive and predictive systems.
However, it is a long and complex journey. It will require collaboration from a wide range of stakeholders, including governments, technology companies, and healthcare providers.
Furthermore, as AI becomes more common, there will be a greater need for security. The world will have to establish global standards to safeguard how AI uses data. In short, the medical and healthcare field has to use AI responsibly.
Have you got any thoughts or project requirements for medical and healthcare? Connect with us today and we’ll take it forward from there.
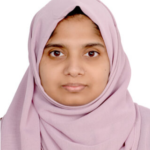
I am a passionate Machine Learning Engineer with 2 Masters in Computer Science – MCA and M Sc (ML /AI). I am proficient in Image Processing using Computer Vision, Machine Learning, and Statistical Modelling Algorithms/Techniques for identifying Patterns and extracting valuable insights.

What to read next
AI in Web Applications: How It Enhances The Online World
AI in Web Applications: How It Enhances The Online World...
Machine Learning in Medical Imaging: Powering Diagnosis
Machine Learning in Medical Imaging: Powering Diagnosis Machine learning is...
Artificial Intelligence for Disabled People: Making A Difference
Artificial Intelligence for Disabled People: Making A Difference AI has...
4 comments on “Machine Learning in Medical: Applications, Advantages & Disadvantages”