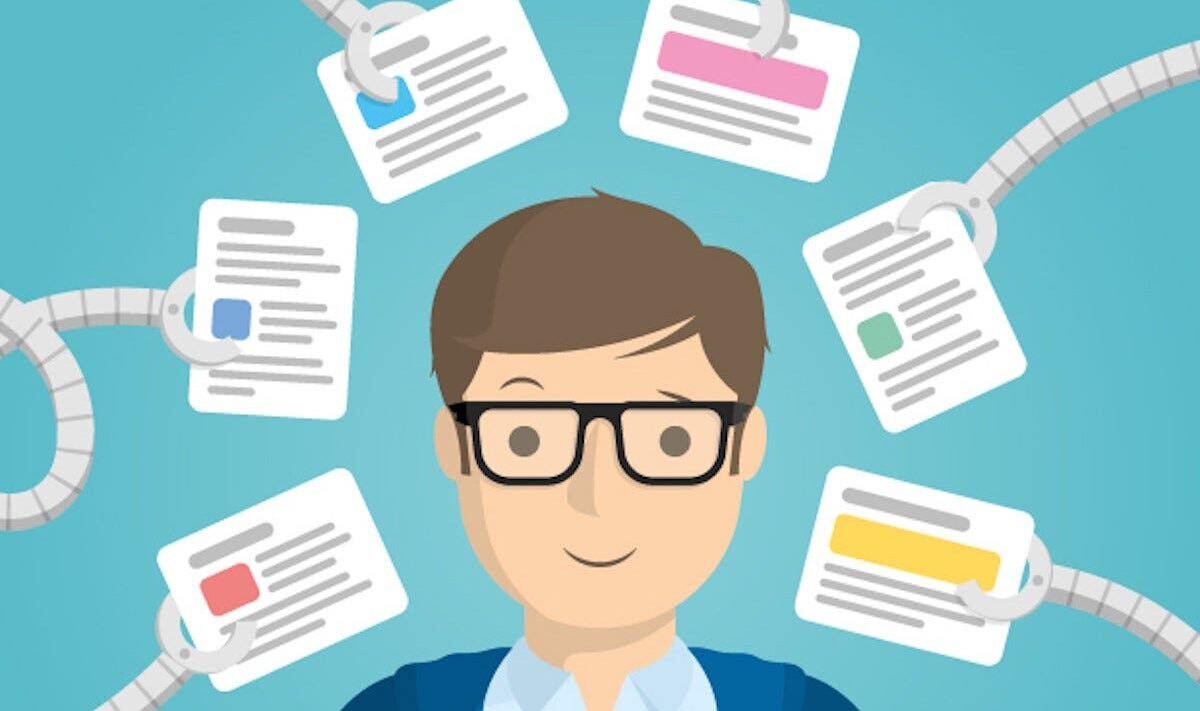
- Rahana A Kadir
- 3
Recommendation Systems: How AI Helps Provide Personalized User Experiences
Recommendation systems are data-filtering algorithms that help online platforms and e-commerce websites provide better suggestions to their customers. It helps them keep their customers engaged and grow their sales by enabling faster discovery. Almost all platforms like YouTube, LinkedIn, Netflix, Spotify, Zomato, etc., employ recommendation systems for their success.
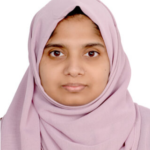
Rahana A Kadir
May 1, 2023 – 5 min read
Recommendation systems are an exceptional application of artificial intelligence (AI) technology. They can suggest items/actions to users based on their interests, past behaviors, or other relevant factors.
Due to the explosion of online shopping and the need to personalize user experiences, recommendation systems gained popularity. However, their application did not stay restricted to e-commerce platforms. Video streaming platforms, music streaming platforms, news websites, advertisements, and e-learning portals also began using them.
As per an estimate, the recommendation systems market will reach $54 billion by 2030. The market size was only $3 billion in 2021, and the forecast is for a CAGR of 37% between the period 2022-2030. With such a promising future, knowing more about recommendation systems is a must.
In this article, we will discuss:
- What are recommendation systems?
- Why recommendation systems are important?
- What are some examples of recommendation systems?
- What are the types of recommendation systems?
- What are the advantages & disadvantages of recommendation systems?
Let’s dive in.
What are Recommendation Systems?
Imagine a scenario where a friend recommends you watch a movie on Netflix. Your sister wants you to watch something else. Your mother wants you to watch her favorite series. Are these recommendations based on what they like or what you like? What if there was someone who understands “your” choice? What if that someone recommends something you want to watch? That’s recommendation systems.
Recommendation systems are automated information filtering systems. They filter some entities composed of machine learning and AI algorithms, helping a customer in discovering what they might like. Thus, saving a lot of time on the user’s end and increasing user retention on the website’s end.
As of today, they are widely used to provide recommendations or suggestions for products, ads, movies, new articles, social media content, TV shows, songs, etc. Apps like Netflix, Amazon, Spotify, Instagram, LinkedIn, and YouTube are all using recommendation systems to serve their users better.
Why Recommendation Systems are Important?
Most companies think they understand their customers. But often customers can behave differently from what businesses think. This is because their choices fluctuate with time. So, it’s important to show them what is relevant to them. It is the need to deliver what matches their choices that make recommendation systems much more important in the online world.
From the customer’s perspective, the recommendation system helps save time. It showcases what interests the user, leading to faster decisions and conversions. From a business perspective, it helps to understand better what the user wants. It also helps to understand what the customer may want in the future.
What are the Types of Recommendation Systems?
Imagine using an online platform for the first time. The moment your sign up, it will ask you for your preference. Ever wondered why? Well, it’s to understand what the user may like and to get a starting point to provide recommendations.
It’s called the cold start problem, where they have no idea of who you are and have no profile for you. Once they know your preferences, your interests, etc. they will start providing you recommendations.
There are two primary types of recommendation systems: content-based and collaborative filtering.
Content-Based Recommendation Systems
Content-based recommendation systems rely on analyzing the attributes of items that a user has liked or interacted with. It is centered on the idea that if a user likes a particular product, they will like similar products.
So, the machine learning algorithm suggests products that are similar to the user’s previous choices. For example, if a person likes horror movies, the system will recommend movies from the same genre. If a person like burgers with cheese, the platform will recommend burgers with cheese and tomato.
Collaborative Filtering Recommendation Systems
Collaborative filtering recommendation systems analyze the behavior of other users with similar preferences and generate recommendations for the user. It focuses on understanding and analyzing the customers’ behavior, preferences, or activities, to provide suggestions based on what others like them like.
So, the machine learning model creates different user profiles, each with a specific set of behavior. Multiple users can have the same user profile. If user A and user B have the same profiles, it will recommend what user A is browsing or viewing to user B and vice versa.
What are Some Examples of Recommendation Systems?
One of the most popular and important examples of recommendation systems is the Netflix Model.
Netflix uses a powerful recommendation system to keep its users hooked to the platform. It is so successful that around 80% of people watch content that comes from Netflix’s recommendation algorithm.
The platform personalizes your experience based on the time you watch Netflix, the device on which you are watching Netflix, your search history or your most recent watch, how long you are watching Netflix in the entire day or in one sitting, etc. In short, these factors are used by machine learning algorithms to understand your persona and take you to the ‘right information’.
Interestingly, the recommendations that Netflix throws at you might be what Netflix threw to other users as well. Why? Because you have matching or similar tastes to those users. All this together, contributes greatly to the success of Netflix.
Besides this, LinkedIn, the largest professional network also employs a recommendation system to grow engagement and generate business. Users get job suggestions based on their skills and past work experience from time to time. If any of the jobs is relevant to them, they go ahead and apply for it. In this way, it helps LinkedIn provide value to its users.
Amazon, the e-commerce giant, is also using a recommendation system to provide product suggestions to users and boost their sales. Similarly, other e-commerce companies are also leveraging the recommendation system for their profit.
What are the Advantages & Disadvantages of Recommendation Systems?
Content-based systems are better suited for recommending niche items. They can also suffer from the “echo chamber” problem. It means that users are only recommended items based on what they already like.
Collaborative filtering systems are better at recommending popular items and can have a bias. It may recommend items that are popular only among a specific demographic.
To overcome these limitations, modern recommendation systems use a combination. They use both content-based and collaborative filtering techniques. They team up with other machine learning algorithms like deep learning models, NLP models, etc., to generate a more accurate recommendation.
However, there are some ethical concerns surrounding the use of recommendation systems. Some critics argue that recommendation systems can create “filter bubbles”. This limits users’ exposure to diverse viewpoints and reinforces existing biases.
There have been concerns that recommendation systems can manipulate users’ behavior or preferences, particularly in the realm of politics. So, R&D is going on to make recommendation systems more transparent and accountable.
For e.g., some systems allow users to see the factors used to generate a recommendation. These systems also allow us to provide feedback on whether a recommendation was helpful. Others are working on developing more diverse and inclusive recommendation algorithms. These algorithms consider a wider range of user preferences and behaviors.
With that said recommendation systems do more good than harm and will stay here for a long time. They are a powerful tool for personalizing user experiences and improving the efficiency of online services. So, sooner or later, we will strike a balance between the benefits and the potential risks.
We at Anubrain have expertise in developing and deploying recommendation systems. If you too wish to integrate a recommendation system into your existing online setup, contact us today.
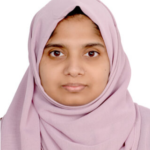
I am a passionate Machine Learning Engineer with 2 Masters in Computer Science – MCA and M Sc (ML /AI). I am proficient in Image Processing using Computer Vision, Machine Learning, and Statistical Modelling Algorithms/Techniques for identifying Patterns and extracting valuable insights.

What to read next
AI in Web Applications: How It Enhances The Online World
AI in Web Applications: How It Enhances The Online World...
Generative AI: An Excerpt Not Generated Using A Machine
Generative AI: An Excerpt Not Generated Using A Machine Generative...
Artificial Intelligence for Disabled People: Making A Difference
Artificial Intelligence for Disabled People: Making A Difference AI has...
3 comments on “Recommendation Systems: How AI Helps Provide Personalized User Experiences”